Why are data hard to value? Data as derived demand
Last Updated November 26, 2018
Nobody wants data just for the sake of data. Data are valued for their end use (derived demand). When data producers and users are the same organization, assessing the value of data is straightforward (primary demand). But when data are shared and put to use by outside organizations (secondary demand), it is much harder to assess their value because producers and hubs often don’t know (1) how the data are used, (2) if the data lead to action, and (3) how demand changes over time.
Primary and secondary demand
To understand why it’s hard to assess the value of data, we need to distinguish between primary and secondary demand (Figure 1). Primary demand occurs when the same organization collects and uses data. For instance, a water treatment plant collects water quality data to inform real-time treatment. Here, the costs to collect data and the impact on water treatment are known and can be monetarily evaluated.
Secondary demand occurs when data are shared with others. The same data can be put to many different uses; however, once data are shared there is not a simple way to know how the data are used. Without knowing the use, we can’t know the value proposition. For instance, downloaded streamflow data may be used for a school project and they may be used to create flood insurance maps. Each end use has very different value propositions.
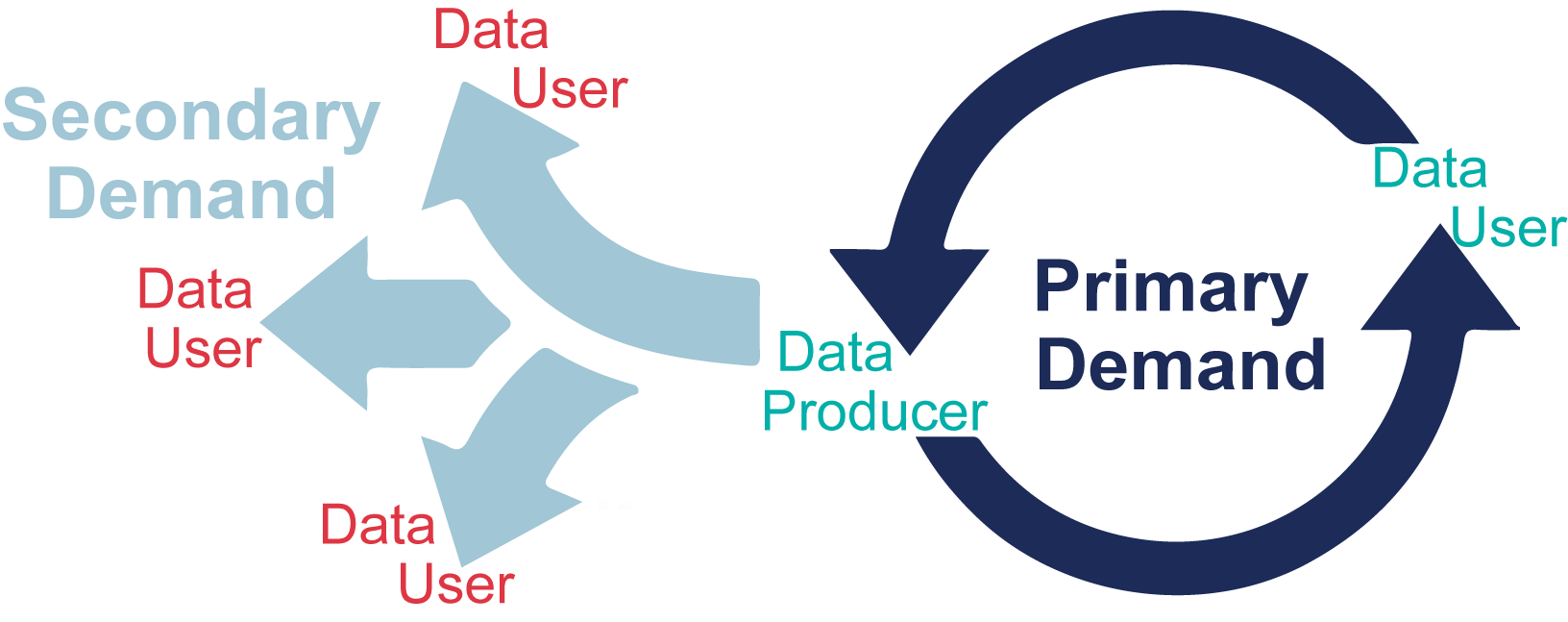
Figure 1: Relation of primary and secondary demand to data producers and users.
Derived demand
Estimating the value of shared data (secondary demand) becomes possible when the data are clearly tied to an end use. This brings us to a third concept, data have derived demand, meaning the data are not desired for themselves, but rather as a means to an end. A helpful analogy are Legos. Neither Lego blocks nor data are desired in and of themselves, but for their end use (Figure 2). Lego blocks are used to create Lego structures for playing while data are used to create information for decision-making. And similar to data, the same Lego block can be used to build any number of structures – a pirate ship or a house or a space station.
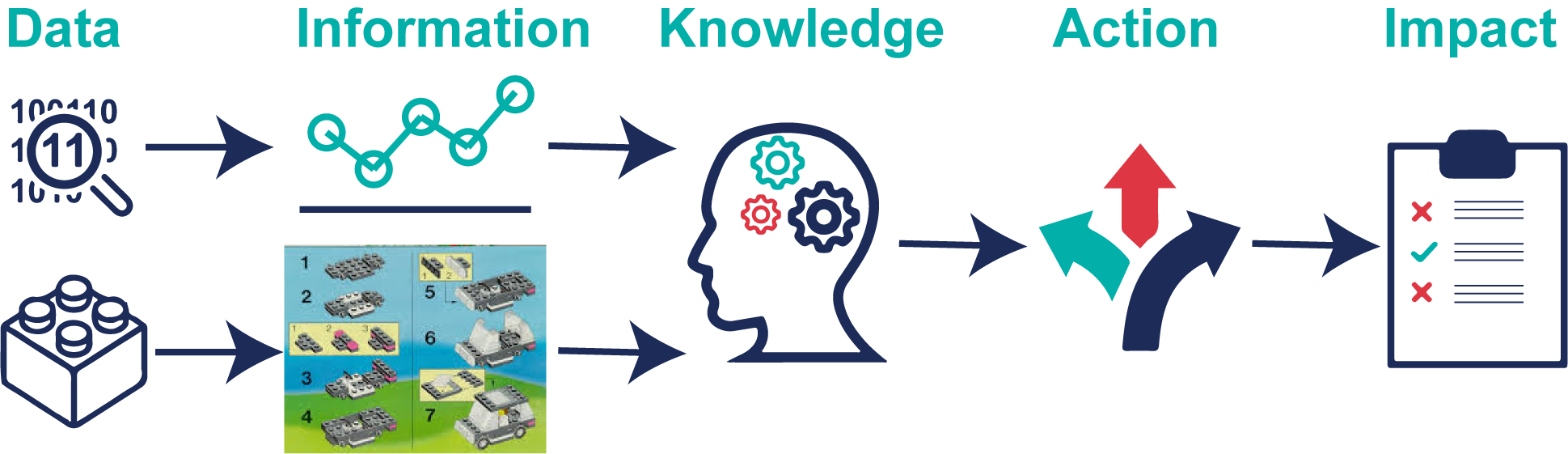
Figure 2: In the same way Lego blocks can be combined to make a variety of Lego structures, data can be combined in different ways to created information for multiple end uses.
Derived demand is hard to economically value because:
- There can be numerous end uses. Just as the same Lego block can be used in a pirate ship or a house, the same precipitation data can be used to inform what you wear today and how much water to use for irrigation.
- Different end uses have different values. The average market value for a single Lego is 10.4 cents, but when Lego blocks are provided in kits, the average value of an individual Lego block changes. For instance, a Lego in a pirate ship kit is worth more than a Lego in a house kit (Figure 3). Similarly, the value of data changes with end use. Precipitation data used for clothing decisions have little long-term economic value, but when used for irrigation decisions they can lead to water savings and increased productivity.
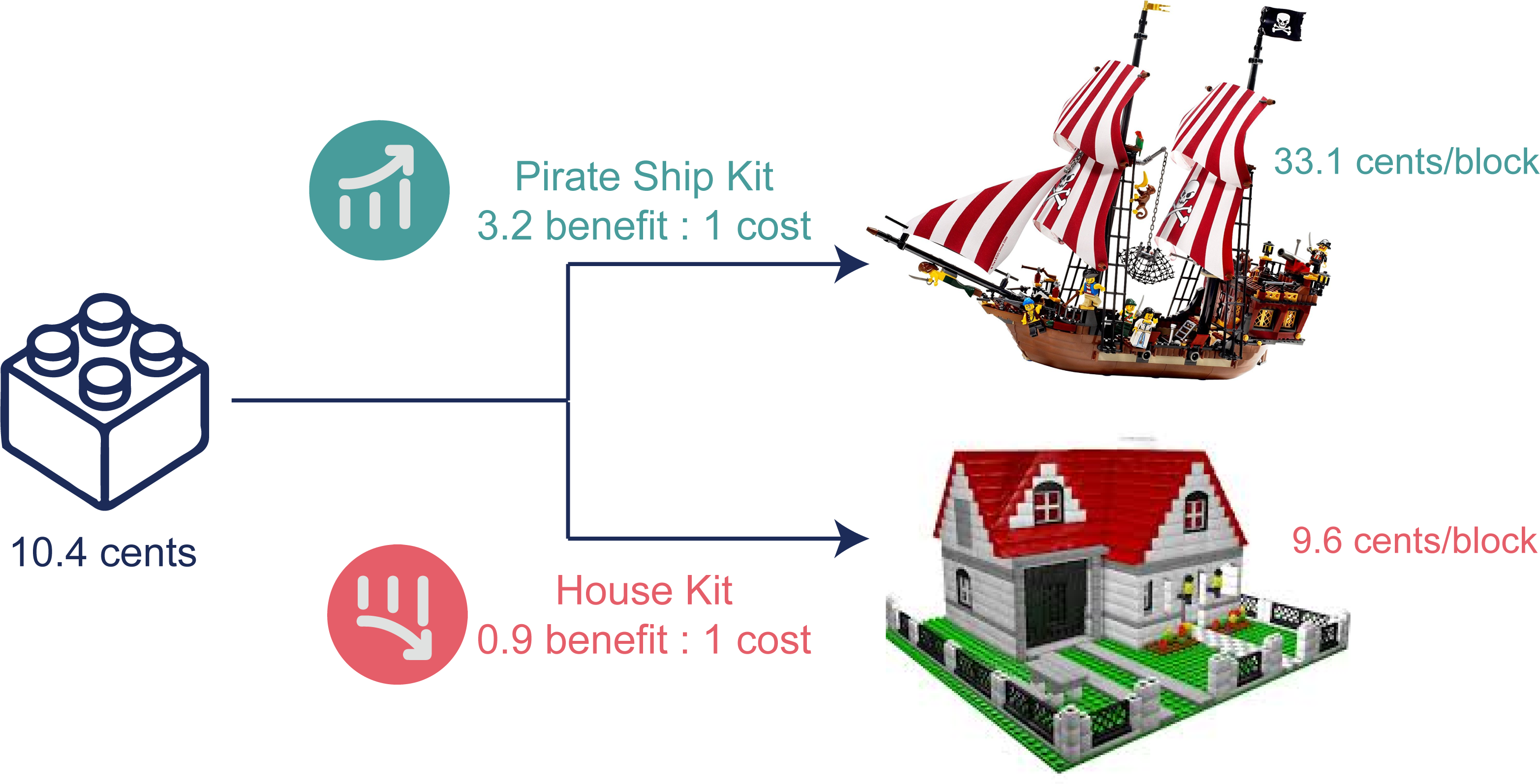
Figure 3: The value of a Lego block changes depending on its end use.
- The value for the same end use changes over time. Today pirate ships are in demand, tomorrow it’s spaceships. Similarly, the value of water data is transient. Precipitation data are more valuable during extremes (flood events or drought) than under normal conditions.
- The end product may not reach the desired impact. You may not end up with a pirate ship because you are missing key pieces or don’t have the knowledge needed to build it. Similarly, precipitation data may not be converted to usable information for irrigation decision-making without temperature, wind speed, crop, and humidity data.
Ensuring data result in a desired impact is key to maximizing their value. A top-down approach that clearly links data to a desired impact can help ensure data have value. These are referred to as use cases, which are like Lego kits. Just as Lego kits overcome design and expertise gaps by ensuring all necessary Lego blocks are present and by providing instructions to reach the desired structure, use cases map how data will become information (what data are needed and how will those data be combined) to inform decision-making.
To have value, data must have impact
Not only is the value of data tied to its end use, it is also tied to the end user. Some end users may be able to fully utilize the data, while other end users may have leverage and execution gaps that prevent the data from having an impact. Let’s look at the following scenarios where mayors of different cities are asked to update floodplain maps.
Tom is the mayor of a city located in a desert. Tom believes updating flood maps will be a huge waste of time and energy. They get little rain and nobody lives near the canyon that occasionally floods. Updating floodplain maps will not impact the city.
Jill is the mayor of a city behind a levee system. She was confused by the call to update floodplain maps because the levees will protect them, and if the floodplain maps indicate the levees won’t protect them, the cost to retrofit the levees is prohibitive. Jill does not have the capacity to take action with updated floodplain maps.
Sam is the mayor of a city whose planners recently called attention to frequent flooding in new areas. Updating floodplain maps would show how the floodplain is changing and could be used to inform rezoning decisions.
These scenarios show the value of data are tied to (Figure 4):
- The strength of the end users prior convictions (can they be swayed by new information).
- The costs of making a wrong decision (deciding how to rezone near a floodplain).
- The capacity to take action based on the information (nobody lives near the floodplain vs affordability of infrastructure upgrades vs deciding whether to rezone the floodplain).
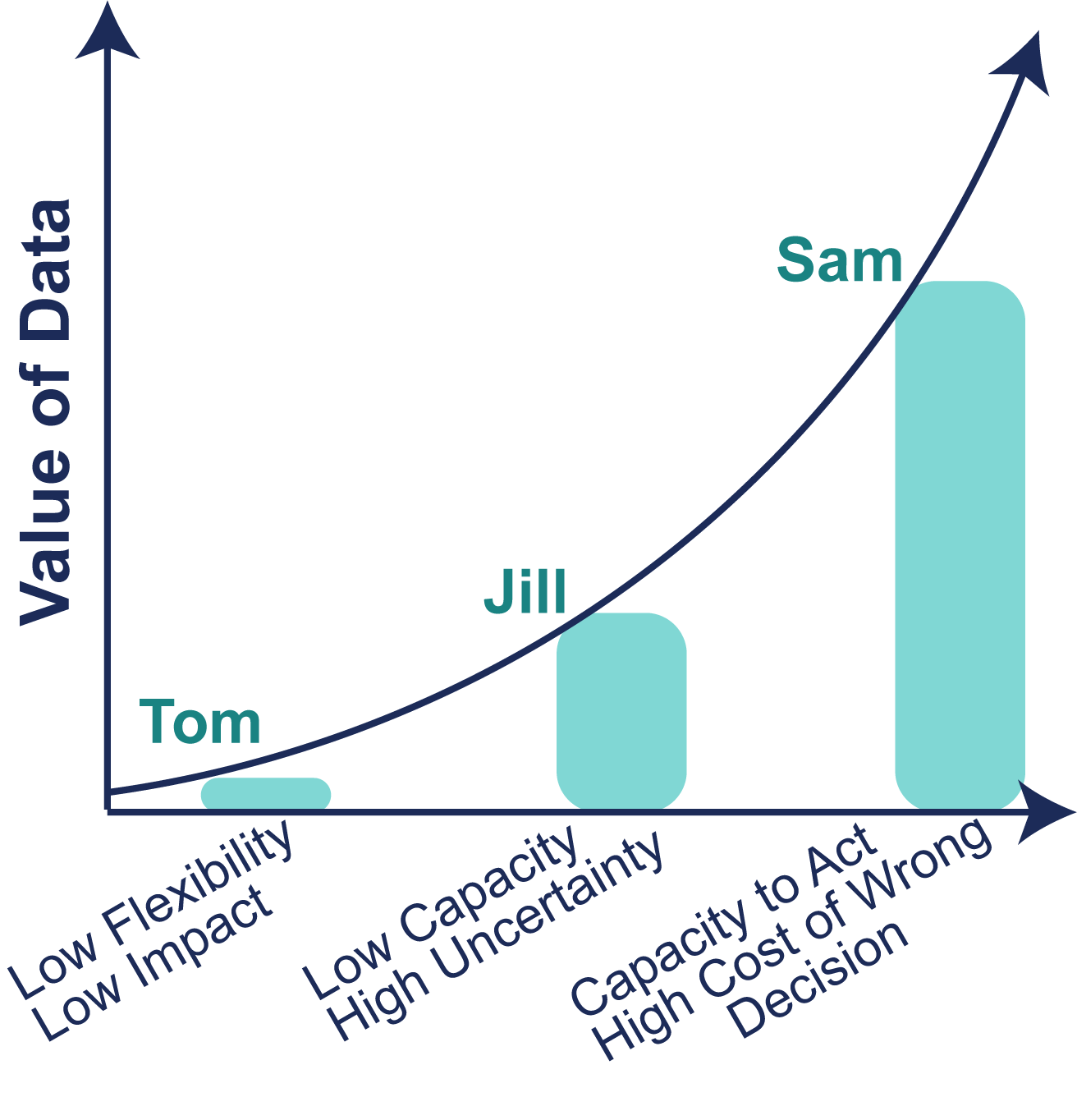
Figure 4: The same data creating the same information have different value depending on the end user.
The plethora of uses and end users makes valuing data put to secondary uses challenging. The value for secondary demand data can be more readily estimated by defining clear use cases (derived demand) for specific end users who have the capacity take action.
For more information:
- Blue Ribbon Task Force. 2010. Sustainable Economics for a Digital Planet: Ensuring Long-term Access to Digital Information.
- Cantor, A. et al. 2018. Data for Water Decision Making: Informing the Implementation of California’s Open and Transparent Water Data Act through Research and Engagement.
- Hamilton, Stu. 2014. Shared Data, Complete with Discoverable Provenance, Have Unbounded Value.