Moving towards valuation by data purpose
Last Updated November 26, 2018
Data are collected for different purposes, shaping their potential value. Here we explore common costs associated with data collection and the impact of different data purposes on its attributes and value.
Organizations track the costs and revenues of providing services in order to inform future investment decisions. We already illustrated the challenges of valuing data because of data’s unique attributes and the different types of demand. Now, we explore how valuing data changes between data producers, hubs, and users, as well as how data’s attributes change based on their primary purpose.
Valuing data by producer, hub, and user
Data producers collect data. Costs for data producers fall into three broad categories: (1) labor to collect, manage, and analyze data, (2) equipment to collect data, and the (3) infrastructure (hardware and software) to store, share, and analyze data. There are upfront capital costs as well as ongoing maintenance and operation costs. The value of the data are realized within the organization (primary demand). However, data producers may share their data with others (secondary demand) (Figure 1).
Data hubs are formalized, structured containers for managing and sharing data. Data hubs ingest data from data producers, and in some instances (such as federal and state agencies) data producers are also hubs. Hubs may not have the cost of producing data, but they do bear costs associated with infrastructure and data management. They may have additional costs to make data interoperable if they collect data from a variety of producers (such as the Water Quality Portal or the National Groundwater Monitoring Network). Hub costs are primarily tied to ensuring data discoverability, accessibility, and usability.
Data users create information and value from data. Costs include resources spent discovering, accessing, and making data usable. The value of data is linked to decisions that lead to impact. Broad categories of impact include:
- monetary (gains or losses),
- loss of life or injury (positive or negative),
- environmental (positive or negative), and
- reputational (market value and public opinion gains or losses).
Not all of value creation is translatable to economic terms and new types of value are continually being created. For instance, who would have anticipated lawyers using evaporation data to assess road friction coefficients in automobile accidents? Who would have thought real estate agents and bankers would invest in water rights data to better inform the future loan risk?
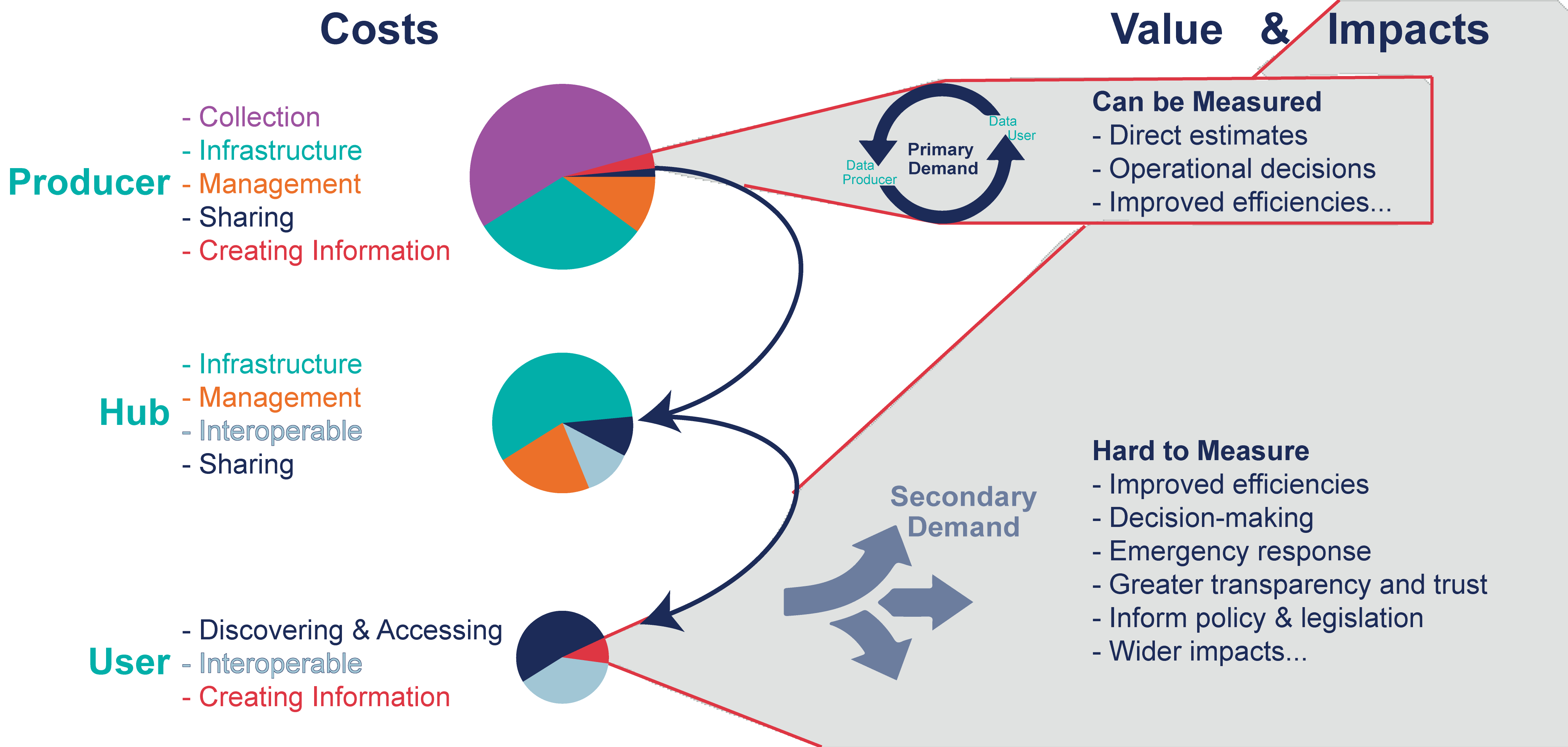
Figure 1: Costs and benefits of data are illustrated by producers, hubs, and users. Examples of common costs and their relative importance are shown in the pie charts. Notice that when data are not shared beyond the producer (primary demand only red box), the value is limited.
Linking data to decisions
There are two general approaches to data collection (Figure 2):
- A bottom-up approach collect as much data as possible and then find ways to use the data. However, this may lead to large volumes of data never being put to use (incurring only costs).
- A top-down approach first identifies the decisions that need to be made, followed by the information needed to make those decisions, and lastly the data needed to generate that information. This ensures that any data collected will be put to use and create value.
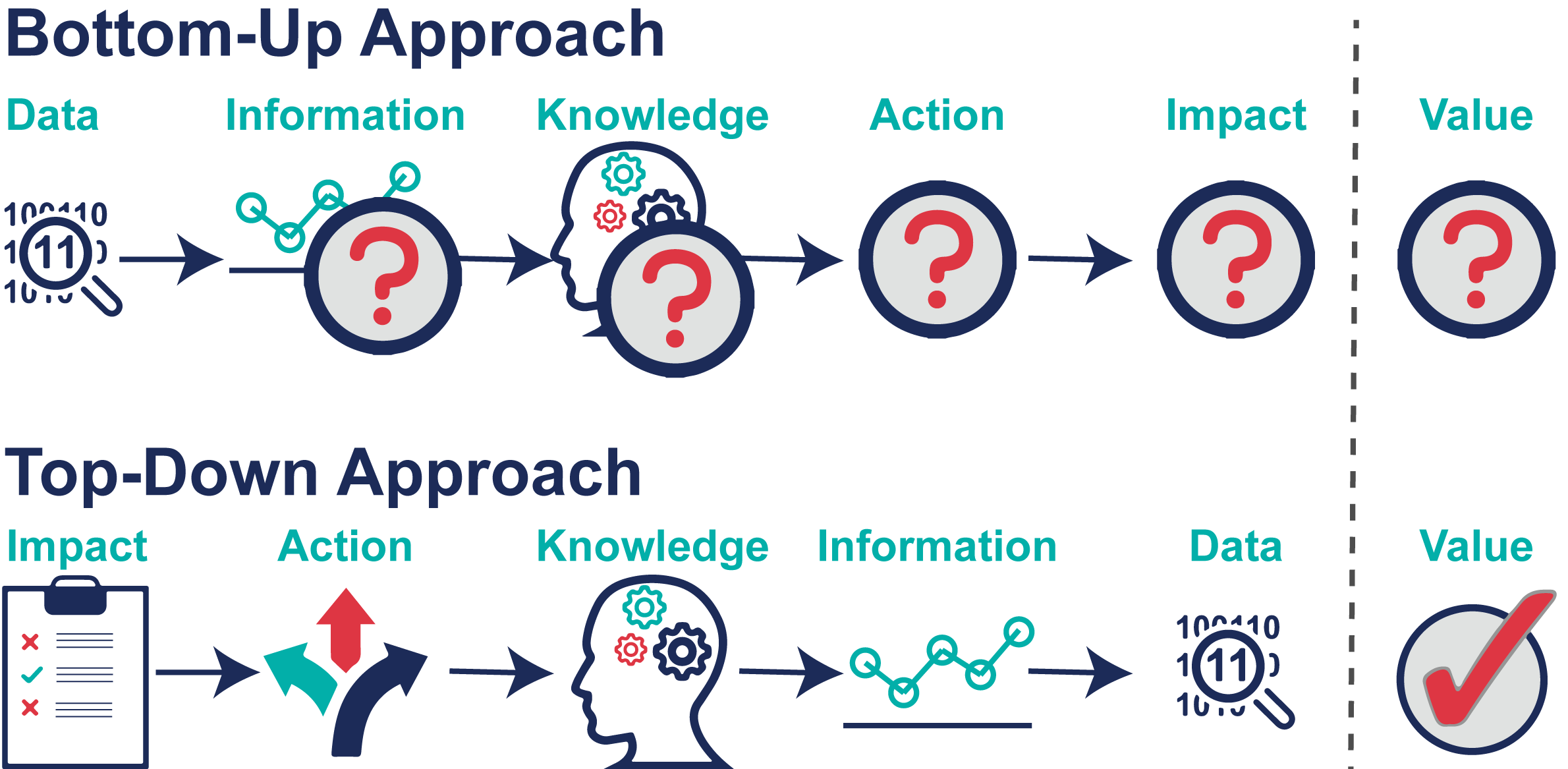
Figure 2: A bottom-up approach collects data first and generates information and knowledge later. A top-down approach develops use cases to inform which data to collect for a particular decision.
Categorizing data purposes
Data collected for different purposes have different attributes. These attributes impact how data are, or can be, put to use. General purpose categories include: operational, broad decision-making, regulatory, and research.
Operational data are used to inform day-to-day operations. These data have the following attributes:
- Pre-determined collection frequency
- Short usable life span
- Time sensitive
- Internal to the organization
- Has value to the organization (data producer)
- Value is quickly realized
In action: Water quality data are used to adjust chemicals treating drinking water.
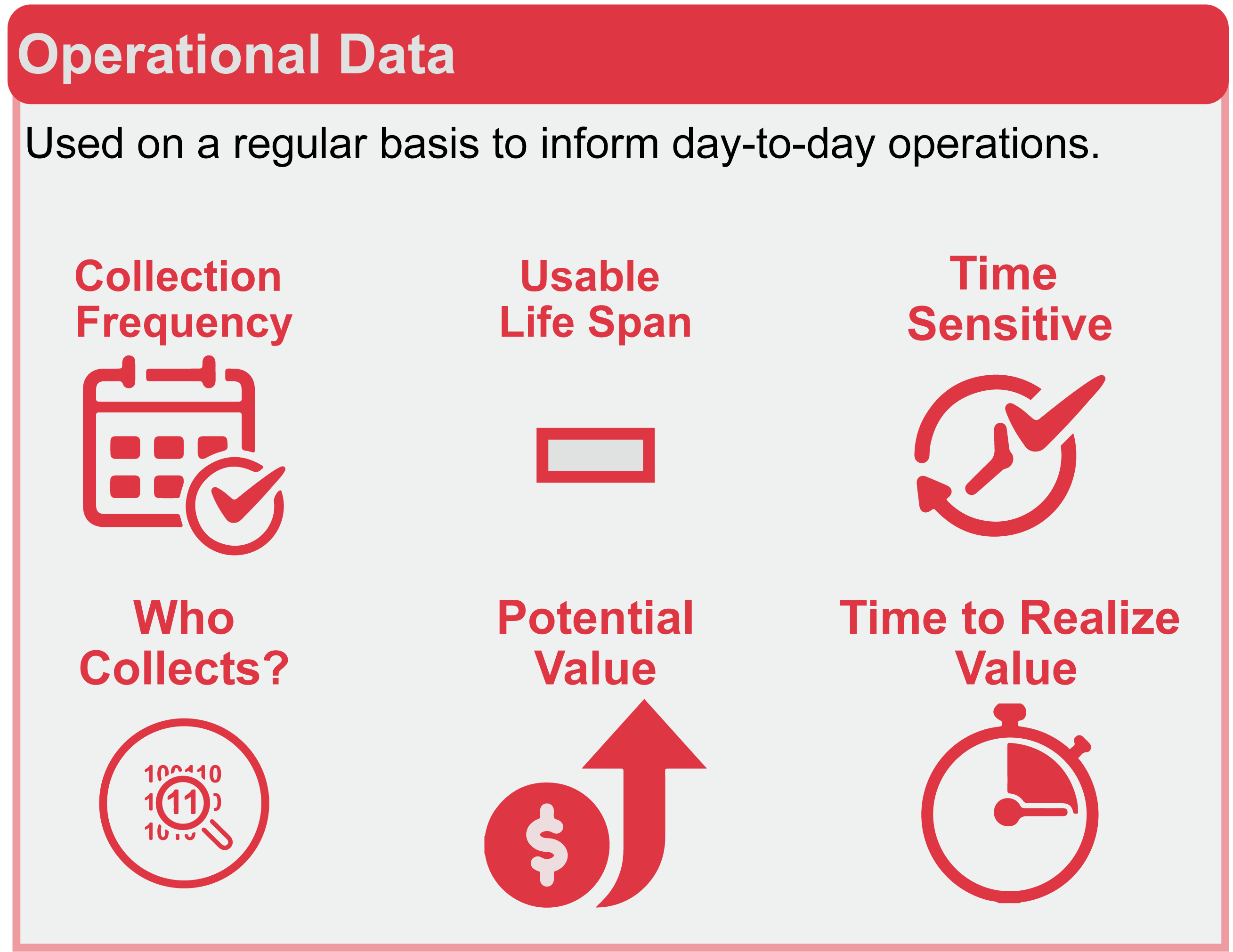
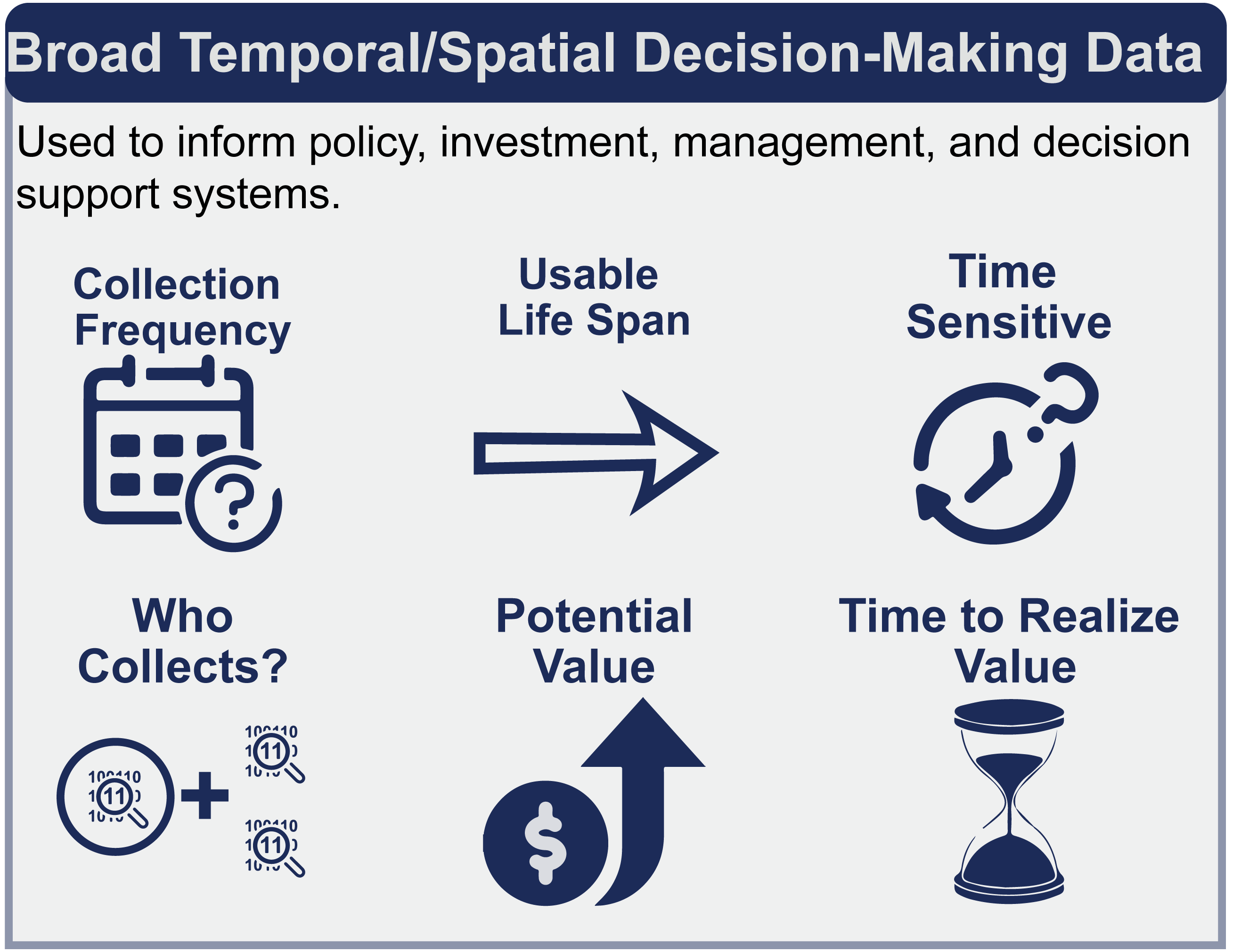
Broad temporal or spatial decision-making data are used to inform policy, investment, management, and other types of decision support systems. The data may be collected within an organization or from outside organizations (such as government monitoring stations or citizen science data). These data have the following attributes:
- No pre-determined collection frequency
- Usable life span varies depending on the decision
- Rarely time sensitive
- Data can be collected internal and external to organization
- Data are valuable to the user regardless of the data producer
- Potential to be of high value
- Value can take a long time to be realized
In action: Developing a water budget to inform decisions on reservoir operations, hydropower generation, and infrastructure investments using data collected by multiple organizations throughout a river basin.
Regulatory data are collected by mandate. Organizations are required to collect and store these data for a pre-defined amount of time. Most of these data are never put to use aside from ensuring compliance and are unlikely to create additional value. These data have the following attributes:
- Pre-determined collection frequency
- Storage is often based on regulation
- Time sensitive
- Internal (and legally required) to the organization
- Cost to collect data often exceeds value to producers (regulators and general public receive value)
- Value is linked to the probability of risk to human or ecosystem health if organization exceeds regulatory limits
In action: Determining whether an industrial discharge into a nearby stream is within regulatory limits to ensure the health of the aquatic environment and downstream water users.
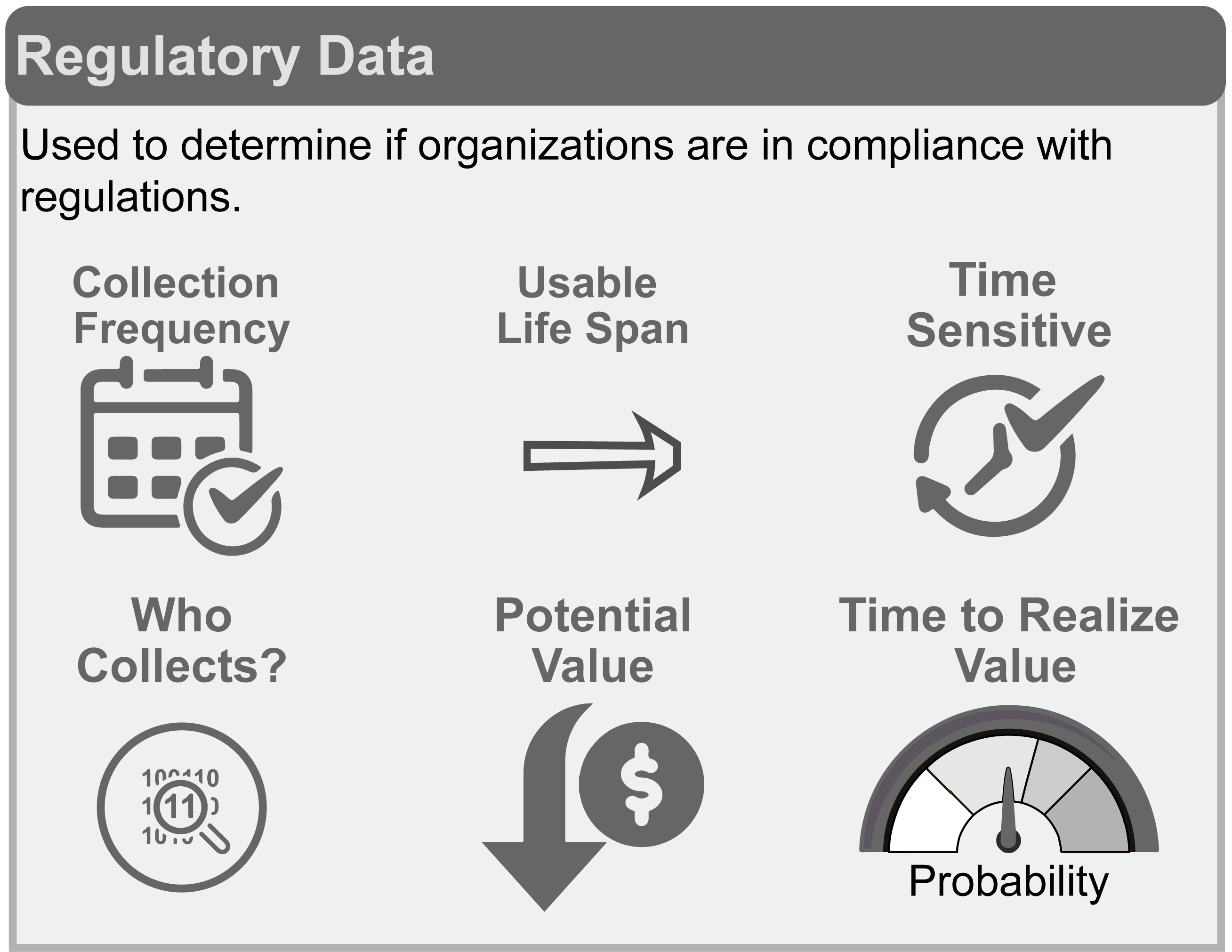
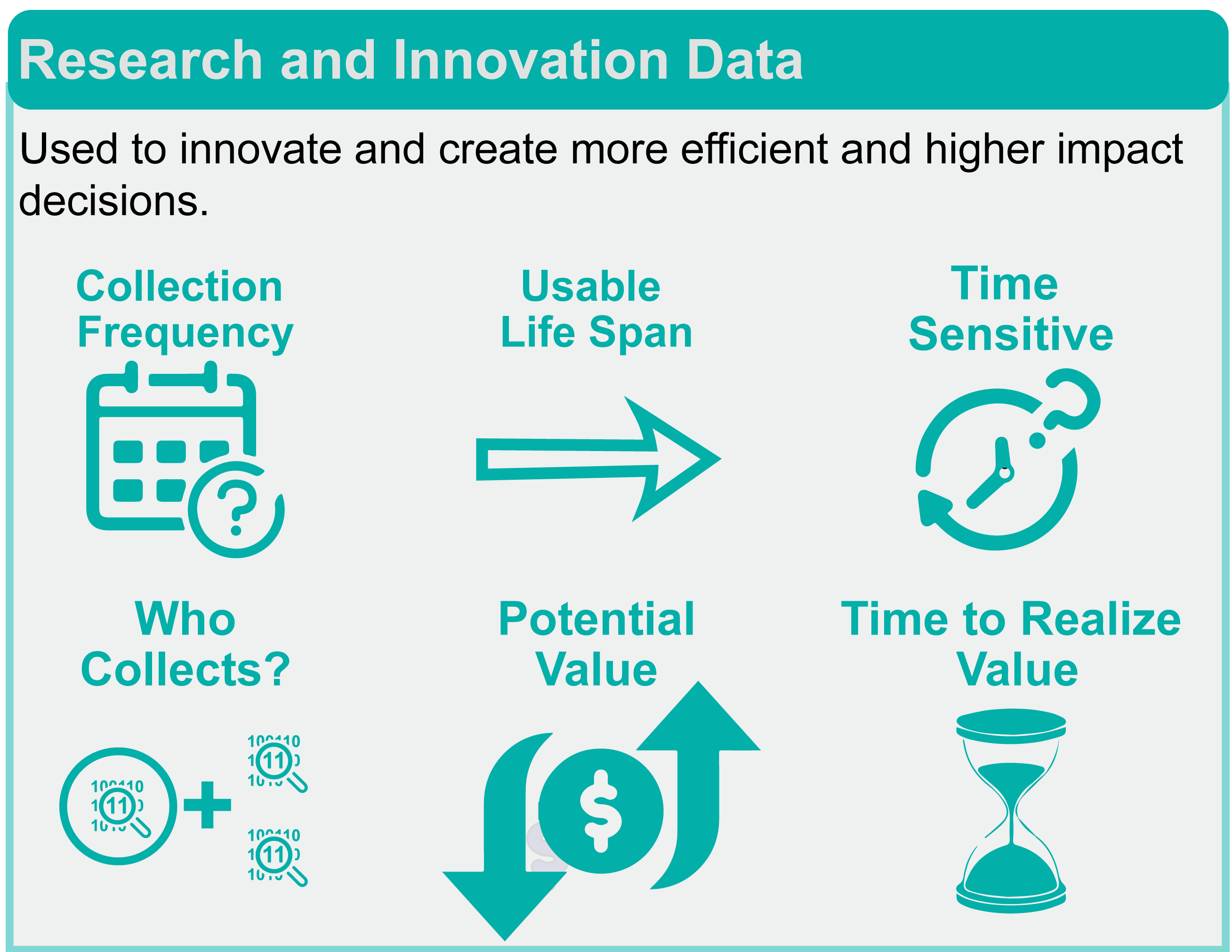
Research and Innovation data are collected to advance knowledge and innovate. The data are high risk (no new insights or information may be derived) but potentially high reward (data may provide great insights that radically improve current decision-making). These data have the following attributes:
- No pre-determined collection frequency
- No pre-determined life span
- Not usually time sensitive
- Data are collected internal to the organization but may be combined with external data
- Often no value will be created, but on occasion there is success and high value reward
- Value takes a long time to be realized
In action: Combining remote sensing data with in-situ water sensors to develop a method for using satellite data to estimate water quality over large regions.
For more information:
- Bergie, N. and J. Houghton. 2014. The Value and Impact of Data Sharing and Curation: A synthesis of three recent studies of UK research data centres.
- Cantor, A. et al. 2018. Data for Water Decision Making: Informing the Implementation of California’s Open and Transparent Water Data Act through Research and Engagement.
- Moody and Walsh. 1999. Measuring the Value of Information: An Asset Valuation Approach. European Conference on Information Systems.
- Stander, J.B. 2015. The Modern Asset: Big Data and Information Valuation.